The Dawning of a Proteomics Era
May 2, 2024 • Jeff Hawkins
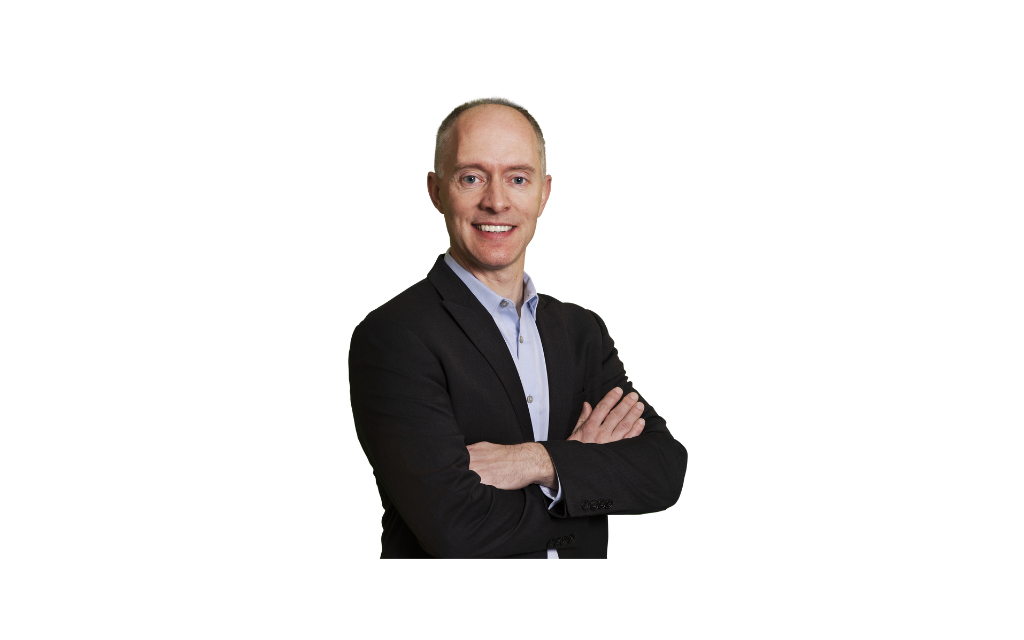
By Jeff Hawkins
The history of scientific research is mostly filled with periods of incremental progress, wherein seemingly disparate studies slowly—and sometimes unknowingly—chip away at conceptual and technological barriers. As these small moments of progress accumulate, they begin to reach a critical mass tipping point, one punctuated by the collapse of barriers and the sudden flood of transformative discoveries. We find ourselves in just such a moment, standing before a crumbling barrier that had long blocked our view into the human proteome.
Over the last decade, we’ve seen significant strides in next-generation sequencing technology which have enabled expansive—and detailed—surveys of the human genetic landscape. However, our ability to understand the molecular nuances that divide health from disease has remained frustratingly limited. A big part of that stems from the fact that, though we can now pinpoint mutations in DNA and RNA with remarkable precision, we often lack the technology to follow those mutations through to the protein level. As a result, there are significant holes in our understanding of the human protein landscape that affect both our understanding of disease and our ability to treat it.
Fortunately, recent technological advances signal a significant cracking of the dam. Improvements to affinity-based and mass-spectrometry (MS) technologies are opening the door to large-scale, high throughput proteomic studies. Simultaneously, next-generation protein sequencing technology has entered the market, enabling researchers to delve deeply into protein sequence variation and cast new light on the dynamics of post-translational regulation. Together, these tools are likely to flood the molecular sciences with proteomic data, revealing novel drug targets and deepening our understanding of human biology along the way.
Proteomics Platforms
Though many researchers are trained to avoid so-called “fishing expeditions”, the past decade has shown us that this sort of large-scale, hypothesis-free data collection can lead to significant breakthroughs. Population genomic studies and national biobanks, for example, have amassed vast troves of whole exome and whole genome sequencing data which is now being used to home in on disease-causing mutations1-3, develop polygenic risk scores4,5, and highlight the breadth of genetic variation6,7. Similarly, whole transcriptome sequencing data enables differential gene expression analyses and the subsequent annotation of complex regulatory networks8.
These studies are only possible because next-generation sequencing technology enables researchers to cast a wide net during data collection. Until recently, researchers have been unable to cast such a net at the protein level, making unbiased proteomics difficult. There are many reasons for this (covered well in these reviews9,10), but the end result is that we know relatively little about the true composition of the human proteome, much less how its dynamics influence disease. Roughly 10% of genome-predicted proteins have yet to be directly observed, while new (and unexpected proteins) continue to surface11,12.
Part of this challenge comes from the fact that the proteome is highly variable in its presentation. With the potential for germline variants, alternative splice forms, and post-translational modifications, the 20,000 genes encoded in the genome are likely to give rise to millions of protein variants, some of which may be differentiated by little more than a phosphorylation mark10.
As yet, there is no way to reliably identify most of these millions of protein variants, much less do so at scale.
Affinity-Based Proteomics Platforms
Affinity-based proteomic technologies use reporter-labeled antibodies or aptamers to identify specific proteins in a given sample or tissue. Traditionally, these technologies have struggled with sensitivity and scale due to the challenges of distinguishing between reporters, as well as the lengthy and non-trivial task of developing a selective binder for each protein variant.
The latter issue has been partially mitigated by synthetic biology which makes it easier to discover and optimize protein binders. Together with miniaturized chemistry and advanced reporter designs (such as the proximity extension of oligomer-bound antibodies), affinity-based technologies have grown from the limited identification of tens of proteins, to now being able to detect thousands, with some studies reporting the identification of up to 4,000 proteins in a single multiplexed assay10,13.
Mass Spectrometry (MS)
At its core, MS works by generating a profile based on charge and weight for each peptide it analyzes. This profile is then used to estimate the peptide’s amino acid composition and subsequent protein identification. Various iterations of the technology can be used for bottom-up or top-down proteomics. Though a gold-standard technology, the use of MS has been severely limited by the high cost of the machinery, as well as the technical expertise required to both run MS experiments and analyze the resulting data.
Nonetheless, workflow improvements have enabled the application of MS in global proteomic studies, with recent publications reporting the reliable detection of nearly 500 proteins in up to 1,000 samples. When focused on casting a wide net and identifying as many proteins as possible, approximately 4,000 proteins can be identified10,14.
The Proteomics Technology Arms Race
That MS and Affinity-based technologies are proving capable of large-scale proteomic studies has led to increased market demand and intense competition among developers. The desire to gain a commercial edge is motivating developers to expand the technologies’ throughput and multiplexing potential. It is likely that, in the next few years, we will see a rapid increase in the breadth of proteomics studies. And, as happened in the genomics space, this will in turn lead to breakthroughs across scientific disciplines.
From Breadth To Depth With Protein Sequencing
Though MS and Affinity-based platforms are growing into ever more important tools for proteomics, they have important limitations when it comes to both cost and their ability to differentiate between similar protein variants. Fortunately, this is where next-generation protein sequencing (NGPS) shines.
NGPS is a new technology that allows for the stepwise annotation of peptides. At present, the only commercially available NGPS system uses kinetic profiles of reporter labeled N-terminal amino acid binders to methodically sequence peptide strands15. This system is designed to be highly sensitive and is capable of identifying a majority of amino acids, as well as many carrying post-translational modifications.
Though the scale of NGPS is currently low, it offers unique advantages over existing technologies. Unlike MS and affinity-based techniques, NGPS requires little technical expertise and is sold at a much lower price point.
These benefits mean that NGPS may be preferred for several applications. First, NGPS can be a powerful tool when researchers need to sensitively and specifically distinguish between nuanced proteoforms. While it may be possible to do this with either MS or protein arrays, the speed, simplicity, and detailed output of NGPS may often be favored. Additionally, comparison of protein identification results from affinity-based and MS technologies suggests that data does not always correlate between the two platforms16. In these instances, researchers may opt to use NGPS as an orthogonal approach to protein identification.
Progress In Motion
Now more than ever, researchers have the ability to track information through life’s central dogma. A commercial arms race is likely to fuel the rapid expansion of proteomics scale, enabling ever more complete characterization of the human proteome and its dynamics during health and disease. Similarly, NGPS technology enables researchers to focus on specific proteins, detailing mechanisms of post-translational regulation and their influence on pathological conditions.
Ultimately these are just tools, each with their own imperfections and needed optimizations. But they are also significant hammers that will help us break through a barrier, one that has long blocked our view of a critical class of biomolecules. Proteins may have been elusive for much of our past, but with these technological advances, we are likely to see a flood of proteomic data in the years to come.
Jeff Hawkins – President and Chief Executive Officer (Bio)
Mr. Hawkins brings over 20 years of experience at the world’s leading life science and diagnostics companies as an accomplished business leader and inventor. Prior to Quantum-Si, he was President and Chief Executive Officer of Truvian Sciences, Inc. where he led the evolution of the company’s benchtop blood testing system from a product concept through technology feasibility and into late-stage development. Prior to Truvian, Mr. Hawkins led the Reproductive and Genetic Health Business Unit at Illumina, Inc., where he oversaw the rapid global growth of next-generation sequencing into new and emerging markets.
During his Illumina tenure, the business unit more than doubled in revenue and established clear market leadership across every major product line and geographic region. Before Illumina, Mr. Hawkins held roles of increasing responsibility across multiple functional areas for GenMark, Hologic, Third Wave Technologies and Abbott Laboratories. Mr. Hawkins holds a B.A. in Chemistry with honors from Concordia University and an MBA from Keller Graduate School of Management. He is co-inventor on 10 issued or pending patents spanning consumables, instrumentation, optics, manufacturing methods and designs.
References
- Conroy, M. C., Lacey, B., Bešević, J., Omiyale, W., Feng, Q., Effingham, M., Sellers, J., Sheard, S., Pancholi, M., Gregory, G., Busby, J., Collins, R., & Allen, N. E. (2022). UK Biobank: a globally important resource for cancer research. British Journal of Cancer, 1–9. https://doi.org/10.1038/s41416-022-02053-5
- Wang, Q., Dhindsa, R. S., Carss, K., Harper, A. R., Nag, A., Tachmazidou, I., Vitsios, D., Deevi, S. V. V., Mackay, A., Muthas, D., Hühn, M., Monkley, S., Olsson, H., Wasilewski, S., Smith, K. R., March, R., Platt, A., Haefliger, C., & Petrovski, S. (2021). Rare variant contribution to human disease in 281,104 UK Biobank exomes. Nature, 597(7877), 527–532. https://doi.org/10.1038/s41586-021-03855-y
- The dual benefits of population-scale genomics. (n.d.). Www.nature.com. Retrieved April 12, 2024, from https://www.nature.com/articles/d42473-019-00374-3
- Kachuri, L., Graff, R. E., Smith-Byrne, K., Meyers, T. J., Rashkin, S. R., Ziv, E., Witte, J. S., & Johansson, M. (2020). Pan-cancer analysis demonstrates that integrating polygenic risk scores with modifiable risk factors improves risk prediction. Nature Communications, 11(1). https://doi.org/10.1038/s41467-020-19600-4
- Mavaddat, N., Michailidou, K., Dennis, J., Lush, M., Fachal, L., Lee, A., Tyrer, J. P., Chen, T.-H., Wang, Q., Bolla, M. K., Yang, X., Adank, M. A., Ahearn, T., Aittomäki, K., Allen, J., Andrulis, I. L., Anton-Culver, H., Antonenkova, N. N., Arndt, V., & Aronson, K. J. (2019). Polygenic Risk Scores for Prediction of Breast Cancer and Breast Cancer Subtypes. The American Journal of Human Genetics, 104(1), 21–34. https://doi.org/10.1016/j.ajhg.2018.11.002
- Bergström, A., McCarthy, S. A., Hui, R., Almarri, M. A., Ayub, Q., Danecek, P., Chen, Y., Felkel, S., Hallast, P., Kamm, J., Blanché, H., Deleuze, J.-F., Cann, H., Mallick, S., Reich, D., Sandhu, M. S., Skoglund, P., Scally, A., Xue, Y., & Durbin, R. (2020). Insights into human genetic variation and population history from 929 diverse genomes. Science, 367(6484). https://doi.org/10.1126/science.aay5012
- Noyvert, B., A Mesut Erzurumluoglu, Dmitriy Drichel, Omland, S., Till, Mueller, S., Lau Sennels, Becker, C., Kantorovich, A., Bartholdy, B. A., Braenne, I., Julio Cesar Bolivar-Lopez, Costas Mistrellides, Belbin, G. M., Li, J. H., Pickrell, J. K., Johann de Jong, Arora, J., Hu, Y., & Wood, C. R. (2023). Imputation of structural variants using a multi-ancestry long-read sequencing panel enables identification of disease associations. MedRxiv (Cold Spring Harbor Laboratory). https://doi.org/10.1101/2023.12.20.23300308
- Costa-Silva, J., Domingues, D., & Lopes, F. M. (2017). RNA-Seq differential expression analysis: An extended review and a software tool. PLOS ONE, 12(12), e0190152. https://doi.org/10.1371/journal.pone.0190152
- Dupree, E. J., Jayathirtha, M., Yorkey, H., Mihasan, M., Petre, B. A., & Darie, C. C. (2020). A Critical Review of Bottom-Up Proteomics: The Good, the Bad, and the Future of This Field. Proteomes, 8(3). https://doi.org/10.3390/proteomes8030014
- Suhre, K., McCarthy, M. I., & Schwenk, J. M. (2020). Genetics meets proteomics: perspectives for large population-based studies. Nature Reviews Genetics, 22(1), 19–37. https://doi.org/10.1038/s41576-020-0268-2
- Omenn, G. S., Lane, L., Lundberg, E. K., Overall, C. M., & Deutsch, E. W. (2017). Progress on the HUPO Draft Human Proteome: 2017 Metrics of the Human Proteome Project. Journal of Proteome Research, 16(12), 4281–4287. https://doi.org/10.1021/acs.jproteome.7b00375
- Valdivia-Francia, F., & Ataman Sendoel. (2024). No country for old methods: new tools for studying microproteins. IScience (Cambridge), 108972–108972. https://doi.org/10.1016/j.isci.2024.108972
- Emilsson, V., Ilkov, M., Lamb, J. R., Finkel, N., Gudmundsson, E. F., Pitts, R., Hoover, H., Gudmundsdottir, V., Horman, S. R., Aspelund, T., Shu, L., Trifonov, V., Sigurdsson, S., Manolescu, A., Zhu, J., Olafsson, Ö., Jakobsdottir, J., Lesley, S. A., To, J., & Zhang, J. (2018). Co-regulatory networks of human serum proteins link genetics to disease. Science, 361(6404), 769–773. https://doi.org/10.1126/science.aaq1327
- Keshishian, H., Burgess, M. W., Gillette, M. A., Mertins, P., Clauser, K. R., Mani, D. R., Kuhn, E. W., Farrell, L. A., Gerszten, R. E., & Carr, S. A. (2015). Multiplexed, Quantitative Workflow for Sensitive Biomarker Discovery in Plasma Yields Novel Candidates for Early Myocardial Injury. Molecular & Cellular Proteomics: MCP, 14(9), 2375–2393. https://doi.org/10.1074/mcp.M114.046813
- Reed, B. D., Meyer, M. J., Abramzon, V., Ad, O., Ad, O., Adcock, P., Ahmad, F. R., Alppay, G., Ball, J. A., Beach, J., Belhachemi, D., Bellofiore, A., Bellos, M., Beltrán, J. F., Betts, A., Bhuiya, M. W., Blacklock, K., Boer, R., Boisvert, D., & Brault, N. D. (2022). Real-time dynamic single-molecule protein sequencing on an integrated semiconductor device. Science, 378(6616), 186–192. https://doi.org/10.1126/science.abo7651
- Eldjarn, G. H., Ferkingstad, E., Lund, S. H., Helgason, H., Magnusson, O. T., Gunnarsdottir, K., Olafsdottir, T. A., Halldorsson, B. V., Olason, P. I., Zink, F., Gudjonsson, S. A., Sveinbjornsson, G., Magnusson, M. I., Helgason, A., Oddsson, A., Halldorsson, G. H., Magnusson, M. K., Saevarsdottir, S., Eiriksdottir, T., & Masson, G. (2023). Large-scale plasma proteomics comparisons through genetics and disease associations. Nature, 622(7982), 348–358. https://doi.org/10.1038/s41586-023-06563-x